|
|
Computer vision, detector training, multiple bounding boxes |
|
MarioSchwedaTH |

Forum-Newbie
|
 |
Beiträge: 1
|
 |
|
 |
Anmeldedatum: 05.08.19
|
 |
|
 |
Wohnort: ---
|
 |
|
 |
Version: ---
|
 |
|
|
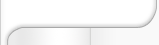 |
|
Verfasst am: 05.08.2019, 10:32
Titel: Computer vision, detector training, multiple bounding boxes
|
 |
|
 |
|
Hello,
I tried to train a detector-neural-network, to detect objects and mark them with bounding boxes.
I tried several parameters (epochs, mini batch size, overlaprange).
But after the training, when I use the detector, the detected objects are marked with multiple bboxes, with slightly different positions, instead with only one bbox.
Do anyone know a reason for this? :-)
Greetings,
Mario
------------------------
Here is the code:
------------------------
%%
clc; clear all;
%% Load Dataset
Dataset=load('imageLabelingSession_Billardkugeln_Gelb_Rot_Weiss_BBoxData.mat'); % Laden der Daten
DataPfade = Dataset.gTruth.DataSource.Source ; % Auslesen der Pfade
DataPfade = cell2table(DataPfade) % Umwandeln in Tabelle
DataBBoxes = Dataset.gTruth.LabelData; % Auslesen der BBoxes
DataTable(:,1) = DataPfade; % Eintragen in gemeinsame Tabelle
DataTable(:,2:4) = DataBBoxes;
DataTable.Properties.VariableNames = {'DataPfade' 'Gelb' 'Rot' 'Weiss'} % Umbennen der Spalten
save('DataTable.mat','DataTable') % Speichern
%%
Bildnummer=randi(height(DataPfade));
I = imread(DataTable.DataPfade{Bildnummer});
BBoxMatrix(1,:) = DataTable.Gelb{Bildnummer};
BBoxMatrix(2,:) = DataTable.Rot{Bildnummer};
BBoxMatrix(3,:) = DataTable.Weiss{Bildnummer}
I2 = insertShape(I, 'Rectangle', BBoxMatrix );
figure(1);
imshowpair(I,I2,'montage');
%%
% Set random seed to ensure example training reproducibility.
rng(0);
% Randomly split data into a training and test set.
shuffledIdx = randperm(height(DataTable));
idx = floor(0.6 * height(DataTable));
trainingData = DataTable(shuffledIdx(1:idx),:);
testData = DataTable(shuffledIdx(idx+1:end),:);
options = trainingOptions('sgdm', ...
'MaxEpochs', 20, ...
'MiniBatchSize', 1, ...
'InitialLearnRate', 1e-3, ...
'CheckpointPath', tempdir);
[detector, info] = trainFasterRCNNObjectDetector(trainingData, 'resnet50', options, ...
'NegativeOverlapRange', [0 0.3], ...
'PositiveOverlapRange', [0.6 1]);
%% Verify the training, run the detector on a test image.
clearvars bboxes
clearvars labels
clearvars scores
clearvars V
clearvars W
Bildnummer=randi(height(testData));
I3 = imread(testData.DataPfade{Bildnummer});
% Run the detector.
[bboxes, scores, labels] = detect(detector, I3);
W=[string(scores) string(labels)];
for i=1:size(labels,1)
V(i,1)=strjoin(W(i,:));
end
V
I3 = insertObjectAnnotation(I3, 'rectangle', bboxes, V);
figure(2)
imshow(I3)
%% Evaluate Detector Using Test Set
% Create a table to hold the bounding boxes, scores, and labels output by
% the detector.
numImages = height(testData);
results = table('Size',[numImages 3],...
'VariableTypes',{'cell','cell','cell'},...
'VariableNames',{'Boxes','Scores','Labels'});
% Run detector on each image in the test set and collect results.
for i = 1:numImages
% Read the image.
I = imread(testData.DataPfade{i});
% Run the detector.
[bboxes, scores, labels] = detect(detector, I);
% Collect the results.
results.Boxes{i} = bboxes;
results.Scores{i} = scores;
results.Labels{i} = labels;
end
% Extract expected bounding box locations from test data.
expectedResults = testData(:, 2:end);
% Evaluate the object detector using average precision metric.
[ap, recall, precision] = evaluateDetectionPrecision(results, expectedResults);
% Plot precision/recall curve
figure(4)
hold on
for i=1:3 %Anzahl Label
plot(recall{i,1}, precision{i,1})
end
xlabel('Recall')
ylabel('Precision')
grid on
title(sprintf('Average Precision = %.2f', ap))
%%
BillardKugelDetektor = detector
save BillardKugelDetektor;
|
|
|
|
|
|
|
Einstellungen und Berechtigungen
|
|
Du kannst Beiträge in dieses Forum schreiben. Du kannst auf Beiträge in diesem Forum antworten. Du kannst deine Beiträge in diesem Forum nicht bearbeiten. Du kannst deine Beiträge in diesem Forum nicht löschen. Du kannst an Umfragen in diesem Forum nicht mitmachen. Du kannst Dateien in diesem Forum posten Du kannst Dateien in diesem Forum herunterladen
|
|
Impressum
| Nutzungsbedingungen
| Datenschutz
| FAQ
| RSS
Hosted by:
Copyright © 2007 - 2025
goMatlab.de | Dies ist keine offizielle Website der Firma The Mathworks
MATLAB, Simulink, Stateflow, Handle Graphics, Real-Time Workshop, SimBiology, SimHydraulics, SimEvents, and xPC TargetBox are registered trademarks and The MathWorks, the L-shaped membrane logo, and Embedded MATLAB are trademarks of The MathWorks, Inc.
|
|